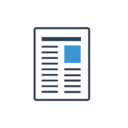
Portraying the expression landscapes of cancer subtypes. A case study of glioblastoma multiforme and prostate cancer


Self-organizing maps (SOM) portray molecular phenotypes with individual resolution. We present an analysis pipeline based on SOM machine learning which allows the comprehensive study of large scale clinical data. The potency of the method is demonstrated in selected applications studying the diversity of gene expression in Glioblastoma Multiforme (GBM) and prostate cancer progression. Our method characterizes relationships between the samples, disentangles the expression patterns into well separated groups of co-regulated genes, extracts their functional contexts using enrichment techniques, and enables the detection of contaminations and outliers in the samples. We found that the four GBM subtypes can be divided into two “localized” and two “intermediate” ones. The localized subtypes are characterized by the antagonistic activation of processes related to immune response and cell division, commonly observed also in other cancers. In contrast, each of the “intermediate” subtypes forms a heterogeneous continuum of expression states linking the “localized” subtypes. Both “intermediate” subtypes are characterized by distinct expression patterns related to translational activity and innate immunity as well as nervous tissue and cell function. We show that SOM portraits provide a comprehensive framework for the description of the diversity of expression landscapes using concepts of molecular function.
DOI: 10.4161/sysb.25897
Projects: GGN - German Glioma Network, HNPCC-Sys - Genomic and transcriptomic heterogeneity of colorectal tumou...
Publication type: Not specified
Journal: Systems Biomedicine
Human Diseases: Brain glioma, Prostate cancer
Citation:
Date Published: 27th Jan 2014
Registered Mode: Not specified

Views: 3481
Created: 10th May 2019 at 12:24
Last updated: 7th Dec 2021 at 17:58

None