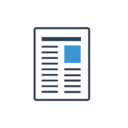
Algorithmic surveillance of ICU patients with acute respiratory distress syndrome (ASIC): protocol for a multicentre stepped-wedge cluster randomised quality improvement strategy

INTRODUCTION: The acute respiratory distress syndrome (ARDS) is a highly relevant entity in critical care with mortality rates of 40%. Despite extensive scientific efforts, outcome-relevant therapeutic measures are still insufficiently practised at the bedside. Thus, there is a clear need to adhere to early diagnosis and sufficient therapy in ARDS, assuring lower mortality and multiple organ failure. METHODS AND ANALYSIS: In this quality improvement strategy (QIS), a decision support system as a mobile application (ASIC app), which uses available clinical real-time data, is implemented to support physicians in timely diagnosis and improvement of adherence to established guidelines in the treatment of ARDS. ASIC is conducted on 31 intensive care units (ICUs) at 8 German university hospitals. It is designed as a multicentre stepped-wedge cluster randomised QIS. ICUs are combined into 12 clusters which are randomised in 12 steps. After preparation (18 months) and a control phase of 8 months for all clusters, the first cluster enters a roll-in phase (3 months) that is followed by the actual QIS phase. The remaining clusters follow in month wise steps. The coprimary key performance indicators (KPIs) consist of the ARDS diagnostic rate and guideline adherence regarding lung-protective ventilation. Secondary KPIs include the prevalence of organ dysfunction within 28 days after diagnosis or ICU discharge, the treatment duration on ICU and the hospital mortality. Furthermore, the user acceptance and usability of new technologies in medicine are examined. To show improvements in healthcare of patients with ARDS, differences in primary and secondary KPIs between control phase and QIS will be tested. ETHICS AND DISSEMINATION: Ethical approval was obtained from the independent Ethics Committee (EC) at the RWTH Aachen Faculty of Medicine (local EC reference number: EK 102/19) and the respective data protection officer in March 2019. The results of the ASIC QIS will be presented at conferences and published in peer-reviewed journals. TRIAL REGISTRATION NUMBER: DRKS00014330.
Projects: SMITH - Smart Medical Information Technology for Healthcare
Publication type: Journal article
Journal: BMJ Open
Publisher: BMJ
Human Diseases: No Human Disease specified
Citation: BMJ Open 11(4):e045589
Date Published: 1st Apr 2021
Registered Mode: imported from a bibtex file

Views: 2094
Created: 24th Feb 2023 at 17:05

This item has not yet been tagged.

None