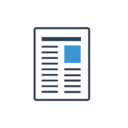
Portraying the Expression Landscapes of B-CellLymphoma-Intuitive Detection of Outlier Samples and of Molecular Subtypes.


We present an analytic framework based on Self-Organizing Map (SOM) machine learning to study large scale patient data sets. The potency of the approach is demonstrated in a case study using gene expression data of more than 200 mature aggressive B-cell lymphoma patients. The method portrays each sample with individual resolution, characterizes the subtypes, disentangles the expression patterns into distinct modules, extracts their functional context using enrichment techniques and enables investigation of the similarity relations between the samples. The method also allows to detect and to correct outliers caused by contaminations. Based on our analysis, we propose a refined classification of B-cell Lymphoma into four molecular subtypes which are characterized by differential functional and clinical characteristics.
Human Diseases: Non-hodgkin lymphoma, B-cell lymphoma
Citation: Biology (Basel). 2013 Dec 2;2(4):1411-37. doi: 10.3390/biology2041411.
Date Published: 2nd Dec 2013
Registered Mode: by PubMed ID

Views: 4237
Created: 10th May 2019 at 11:32
Last updated: 7th Dec 2021 at 17:58

None