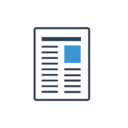
Reduction of platelet outdating and shortage by forecasting demand with statistical learning and deep neural networks: Modeling study

BACKGROUND: Platelets are a valuable and perishable blood product. Managing platelet inventory is a demanding task because of short shelf lives and high variation in daily platelet use patterns. Predicting platelet demand is a promising step toward avoiding obsolescence and shortages and ensuring optimal care. OBJECTIVE: The aim of this study is to forecast platelet demand for a given hospital using both a statistical model and a deep neural network. In addition, we aim to calculate the possible reduction in waste and shortage of platelets using said predictions in a retrospective simulation of the platelet inventory. METHODS: Predictions of daily platelet demand were made by a least absolute shrinkage and selection operator (LASSO) model and a recurrent neural network (RNN) with long short-term memory (LSTM). Both models used the same set of 81 clinical features. Predictions were passed to a simulation of the blood inventory to calculate the possible reduction in waste and shortage as compared with historical data. RESULTS: From January 1, 2008, to December 31, 2018, the waste and shortage rates for platelets were 10.1% and 6.5%, respectively. In simulations of platelet inventory, waste could be lowered to 4.9% with the LASSO and 5% with the RNN, whereas shortages were 2.1% and 1.7% with the LASSO and RNN, respectively. Daily predictions of platelet demand for the next 2 days had mean absolute percent errors of 25.5% (95% CI 24.6%-26.6%) with the LASSO and 26.3% (95% CI 25.3%-27.4%) with the LSTM (P=.01). Predictions for the next 4 days had mean absolute percent errors of 18.1% (95% CI 17.6%-18.6%) with the LASSO and 19.2% (95% CI 18.6%-19.8%) with the LSTM (P<.001 both models allow for predictions of platelet demand with similar and sufficient accuracy to significantly reduce waste shortage in a retrospective simulation study. the possible improvements inventory management are roughly equivalent us per year. conclusions:=""></.001>
Projects: SMITH - Smart Medical Information Technology for Healthcare
Publication type: Journal article
Journal: JMIR Med. Inform.
Publisher: JMIR Publications Inc.
Human Diseases: No Human Disease specified
Citation: JMIR Med. Inform. 10(2):e29978
Date Published: 1st Feb 2022
Registered Mode: imported from a bibtex file

Views: 1110
Created: 24th Feb 2023 at 17:05

This item has not yet been tagged.

None